Data
Jun 22, 2020
Asset Reliability & Maintenance in the Oil and Gas Industry Part 2: Optimized Maintenance Through Big Data Analytics
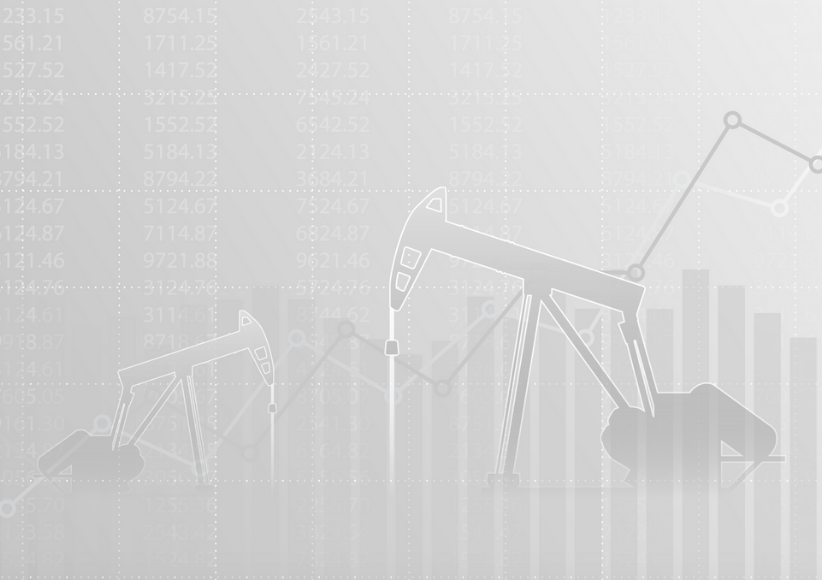
Oil and gas companies can make better maintenance decisions by leveraging the right data and utilizing optimized models to improve reliability. Overcoming the technical hurdles required to improve data quality and implement these optimized models can boost efficiency and save money, making them worthy investments for most companies.
In part one of this series, we introduced the different asset reliability and maintenance strategies, displayed in the graph below, which communicate the difference between traditional industry standards and more optimized models. We also discussed the benefits of becoming data-driven, like increasing savings of time and money, improving safety, and creating a competitive advantage. In this post, we will look at the three optimized models and how to leverage data appropriately to move toward strategic maintenance.
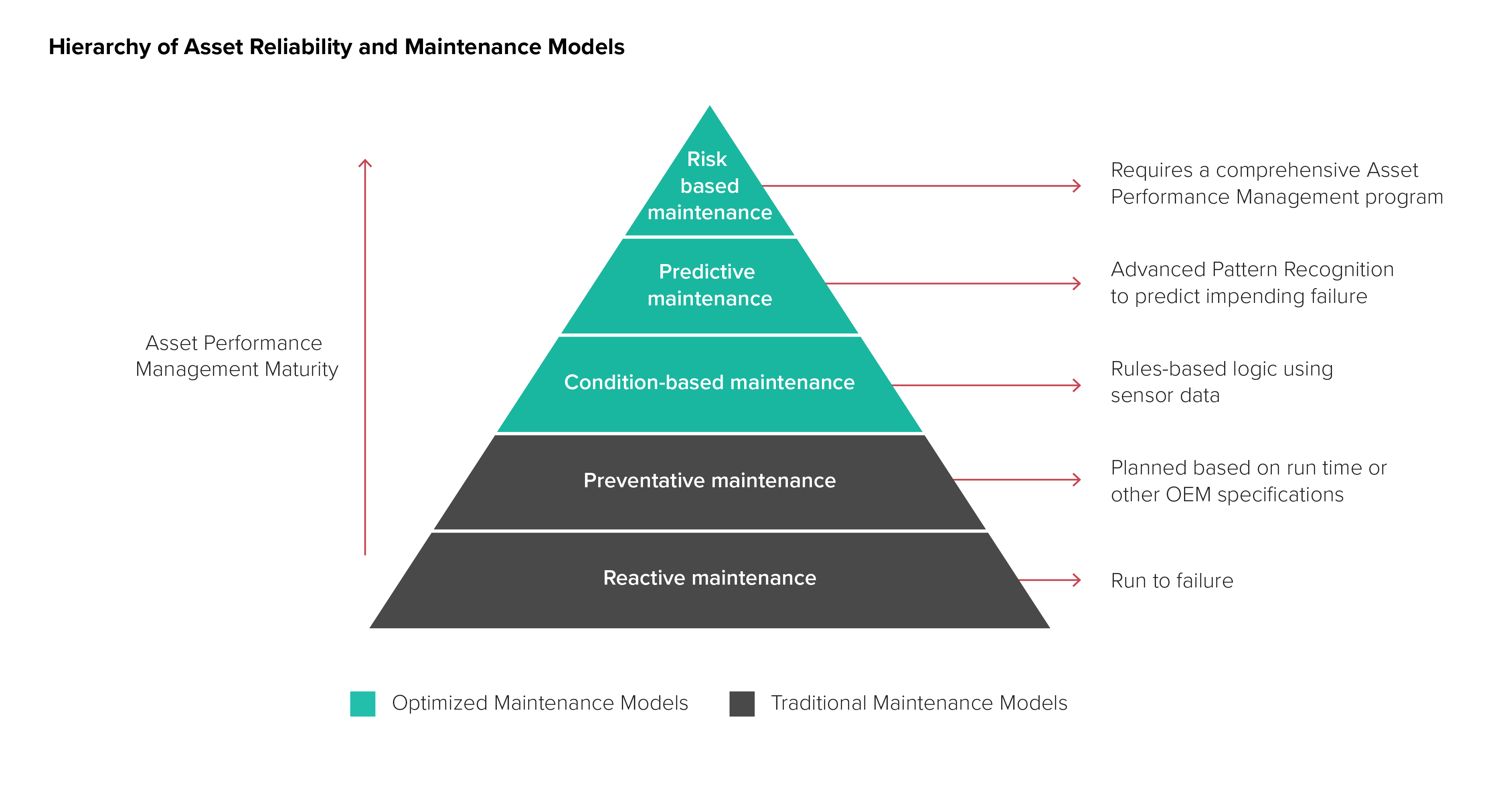
In order to improve the process of asset reliability and maintenance, companies can call upon data and advanced analytics to develop a strategy that balances saving money and operating as safely and efficiently as possible. This allows companies to make the switch from traditional to optimized models. It’s important to note that these strategies are not mutually exclusive and can be used in different contexts or even as a hybrid. There are many ways digital processes can help, from simply adding sensors to equipment to analyzing robust streams of data to predict failures.
The “Optimized” Models
The top three levels of the pyramid—condition-based, predictive, and risk-based—rely heavily on data to ensure that equipment is maintained and repaired at the right time to prevent failures and unnecessary spending. These strategies represent maturity and should not be reviewed in isolation of each other.
Condition-Based Maintenance
Condition-based maintenance defines acceptable operating conditions and implements frequent checkpoints to determine the condition of the asset, which could include visual inspections, tests, performance data, etc., to estimate the optimal time for maintenance. Companies may install sensors on equipment that collect data for measuring the condition or performance of the asset, which can eliminate the need for continuous manual inspections. That data is then aggregated into dashboards for better understanding. The goal of condition-based maintenance is to schedule the maintenance just before failure, which requires technicians to rely on the available data to understand the state of the asset at any given time. This decreases the likelihood of unplanned shutdowns and increases productivity of the asset.
Predictive Maintenance
An even more sophisticated approach is predictive maintenance, which combines the condition-based diagnostics with predictive algorithms and machine learning (ML) to forecast the exact point of failure. The timing of maintenance is therefore much more precise and reaps higher rewards in terms of efficiency and savings.
Risk-Based Maintenance
Risk-based maintenance requires companies to right size their assets. Certain assets are more critical to the business and thus pose a higher financial and operational risk when they fail. These assets should be maintained more often and with more precise care. Other assets are of lower risk and can be maintained suboptimally to recognize cost savings and greater efficiencies. Utilizing different maintenance strategies depending on the value of the asset to the business will optimize operations and cost.
A Lagging Industry
In order to implement condition-based, predictive, and/or risk-based maintenance models, companies must properly leverage their data, an undertaking that many oil and gas companies have struggled to do in the past. This has historically been because of the inability to access data, poor data quality, and low computing power. Today, increased access to data, improved data quality, and high computing power along with digital innovation has made it possible—and somewhat a necessity—to be data-driven. Many oil and gas companies have automated key processes and increased operational efficiency by leveraging data, but there has been an industry-wide lag in optimizing asset reliability and maintenance strategies. Reactive and preventative models are still considered industry standard.
A Global Digital IQ Survey conducted in 2018 indicates the top reasons the oil and gas industry is slow to change are inflexible/slow processes and outdated technology. The vitality of safety forces change to occur at a measured pace, meaning if a company’s culture has been centered around reactive processes and “firefighting” in the past, it will not be easy to transition to a culture of proactivity and digital innovation. Furthermore, outdated technology and equipment lead to an increased effort to fix errors that may not have occurred with modern assets—or better data.
Leveraging Data Appropriately for Improved Asset Reliability and Maintenance
When companies wish to become more data focused and reap the rewards of improved data, a natural first step is to begin gathering data and find a way to house it. There are two common data repositories—data lakes typically store large amounts of unstructured data, while data warehouses exist for reporting and analysis and therefore require front-end cleansing. A more detailed comparison of the two can be found in this post, written by data and analytics expert Tyler Estes.
Both repositories have a part to play in asset reliability and maintenance, but with the influx of large volumes of data, companies need a data lake above all else. A data lake is ideal for incorporating internal and external data in any format, which will allow companies to build the necessary forecasting models for maintenance predictions.
However, collecting large amounts of data without making it useable can turn the data lake into a data swamp where there’s no organized structure or active management. Once the data lake is functional, effort will be required to deliver the right information at the right time to the right people. This is an investment of time and money, which makes it a risky undertaking for some companies.
Credera always begins any initiative with a current state assessment. Determining the state of people, process, and technology through the lens of optimizing asset reliability and maintenance would most likely lead to recommendations surrounding data cleansing and ongoing governance. Once the data is cleansed and updated timely, implementing optimized models can become a reality.
The Case for Improving Efficiencies
Now more than ever, with the worst operating conditions and macroeconomic headwinds the industry has seen in the last 20 years, companies are dealing with lower margins and need to reap cost savings wherever they can. Improving operational efficiencies by investing in data now will have unmatched, lasting benefits, one of which will be the ability to survive such market hostility.
If you are interested in learning more about optimized asset reliability, maintenance models, and how to leverage data appropriately for implementation, email us at findoutmore@credera.com.
Also check out part three of this series, which provides a deeper understanding of each optimized model and how to determine which is right for you.
Contact Us
Ready to achieve your vision? We're here to help.
We'd love to start a conversation. Fill out the form and we'll connect you with the right person.
Searching for a new career?
View job openings