Data
Oct 30, 2020
Asset Reliability & Maintenance in the Oil and Gas Industry Part 4: 3 Steps to Optimized Maintenance
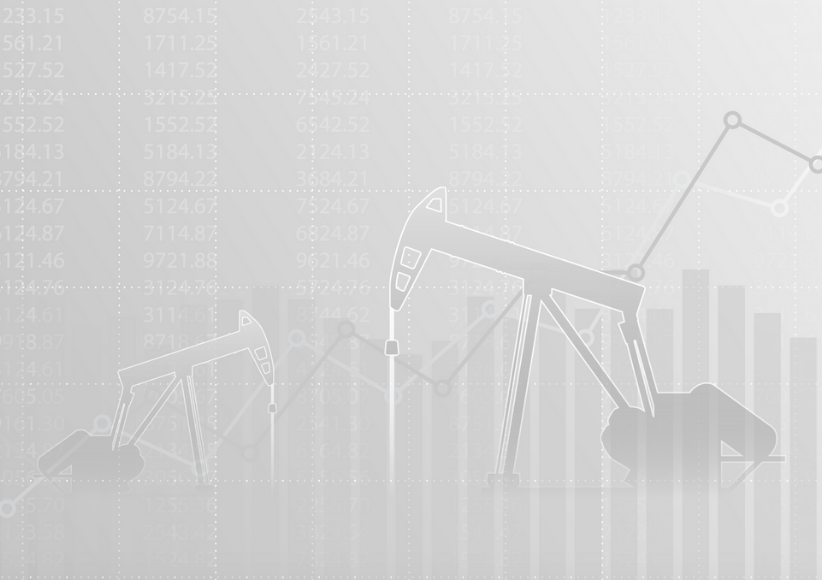
In parts one, two, and three of this series, we looked at the benefits of optimizing operations through data, specifically in asset reliability and maintenance, which included saving time and money, improving safety, and creating a competitive advantage. We also reviewed the optimized maintenance models in detail, including condition-based, predictive, and risk-based maintenance (demonstrated in the graphic below) and how to choose which one is right for an organization. In this post, we’re going to discuss the standard process to make sure your organization is ready for the higher levels of optimized maintenance.
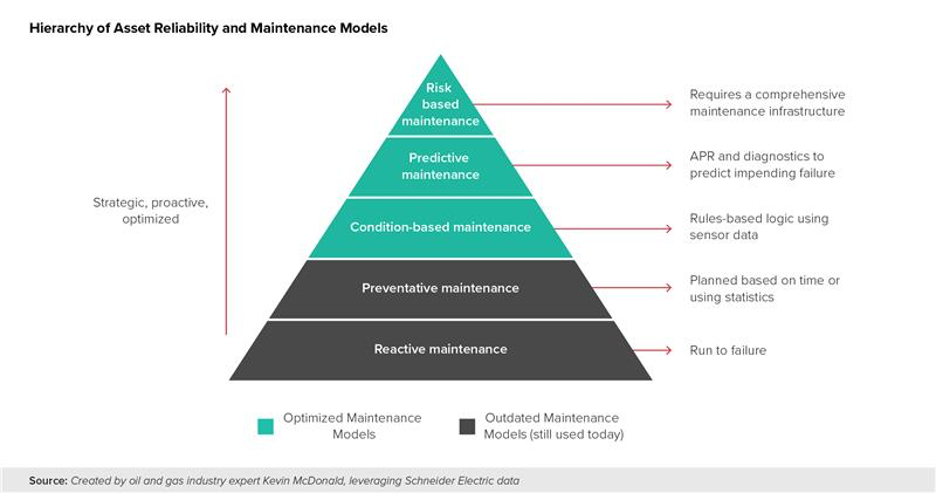
Lay the Groundwork for Optimization
Typically, optimizing maintenance happens in a maturity model approach implemented through crawl, walk, and then run phases, or starting small and improving or adding along the way. Once an organization chooses a maintenance model, it can be tempting to skip to the run phase right away. This would look like installing sensors and setting up machine learning (ML) algorithms, which are subsets of artificial intelligence. Sensors track the equipment data and the ML algorithms read the data and improve over time to provide recommendations of when to conduct maintenance with optimal timing.
It is vital, however, to start with crawl or walk depending on where the organization is on the maturity model. This means many organizations will complete some data assessment and cleanup first. Just like in a landscaping project, you wouldn’t plant new trees and flowers without first assessing the grounds and ridding the property of any weeds. In this scenario, those weeds would be the inconsistencies, errors, and gaps in an organization’s dataset that could cause issues when trying to make data-driven decisions.
Since each organization is different and each maintenance and ML model would require a tailored implementation, we’ll outline the three steps in the standard process of “getting the weeds out of the garden” before organizations can begin monitoring the health of their assets and reaping the benefits of optimized maintenance using ML. The process starts with a current state assessment to better understand what cleanup activities need to occur. Master data management (MDM) activities, or the actions taken to create source-of-truth data upon which ML algorithms can work, are then performed to clean up and prepare the data. After these activities, organizations can then implement ML algorithms to support their chosen maintenance model and ensure the data remains clean and usable. For more details about implementing machine learning, see this post.
Step 1: Initial Assessment of People, Process, and Technology
When we conduct initial state assessments of an organization’s datasets, we also take the pulse of the data landscape of an organization. We not only determine how accurate, clean, and reliable the data is, but also if data and reporting are seen as necessary evils or chances to optimize operations. This process is called data profiling.
Clean and healthy data will lay the foundation for optimized maintenance models. To find success, employees should have buy-in and education and the processes should support ongoing, proper maintenance of the data to increase the chances of successful adoption. The initial assessment will inform Credera of the data cleansing activities that need to occur as well as any culture or process opportunities or changes that will enhance the implementation efforts.
We’ve helped many organizations through a current state assessment by comparing the maturity of a company’s dataset against Credera’s Artificial Intelligence (AI) Maturity Model (see diagram below), created using Gartner’s analytic continuum. Data science experts can do a brief discovery phase and pinpoint where an organization falls on the model, giving a clear picture of the path forward.
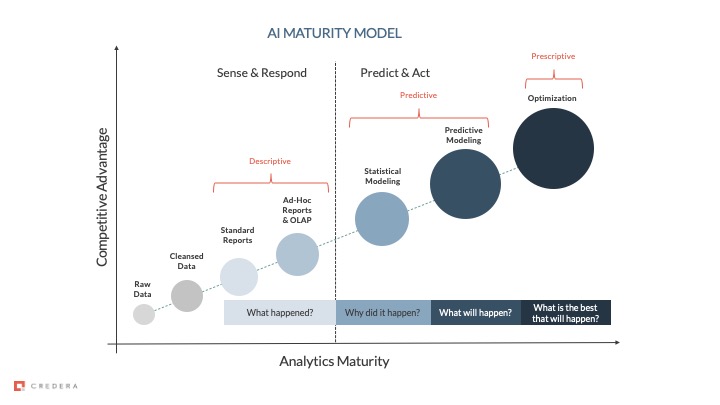
Credera AI Maturity Model (Source: Credera leveraging Gartner’s analytic continuum)
Step 2: Master Data Management Activities & ML Proof of Concept
Next, it is important to act upon the findings from the current state assessment, which will ensure an organization’s data and culture are properly suited to take on advanced analytics.
A visual representation of the role master data plays in an organization can be found below. The key callout is that master data merges all the data sources to provide a source of truth for all reporting and analytics. Determining the optimal time to conduct maintenance relies on proper reporting, displaying the importance of data that organizations can rely on.
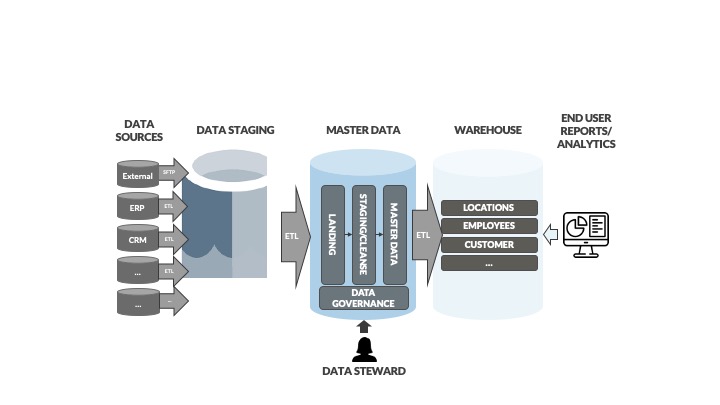
The role of master data in the data ecosystem (Source: Credera’s Data and Analytics Practice)
Credera has seen organizations that need a wide variety of data activities, and a common pattern among many is a struggle to make data a priority. This leads to inaccurate and inconsistent datasets, which creates long-term issues, if not also immediate negative impacts. Some examples of master data management (MDM) activities to rectify these issues are data cleansing to remediate immediate issues and a governance plan to ensure long-term data health.
Data cleansing requires manually improving each dataset to generate correct and consistent data, which also includes eliminating irrelevant data. A data governance plan sets up the rules and regulations for data entry and management, which will help keep the data accurate and consistent going forward. Credera created a data governance plan for Loop, an oil and gas company based in Louisiana. We partnered with Loop to determine which fields in each data set were necessary for moving forward and how each one should be regulated.
Along with data improvements, there are also many people and process opportunities that have been a critical part of success. A common example is the implementation of a steering committee and conducting proper training to ensure data is prioritized and issues are raised and discussed with the proper attention. In this step, develop an ML proof of concept (POC) using pre-defined criteria for success. This POC will transform over time as new information is gathered and will eventually become the final model that is leveraged by an organization. For more information on the ML proof of concept and how it is created, please see this post.
Step 3: Implementation of ML and Desired Maintenance Model
After completing the appropriate MDM activities, the data is now prepared to take on advanced analytics (the autonomous or semi-autonomous examination of data) to support optimized asset reliability and maintenance. Organizations will certainly benefit from an ML algorithm to support their chosen maintenance model, so alongside implementing sensors and monitoring the health of their assets, organizations can build an ML model to understand the data.
Once the algorithm is in place, organizations can then accurately monitor their assets and conduct maintenance at optimal times, delivering numerous benefits such as improved safety, decreased down time, and monetary savings.
Leverage Advanced Analytics for Your Organization
There are three types of models that will optimize asset reliability and maintenance for most organizations; condition-based, predictive, and risk-based maintenance all call upon data to inform organizations of the most optimal timing for asset maintenance. It is vital to first understand the current state of an organization’s data to then clean it up and prepare it for reporting and decision making.
Improving an organization’s dataset without addressing people and process will decrease the likelihood of successful implementation and adoption, since the ongoing maintenance will not be supported. Therefore, addressing all facets of people, process, and technology are highly recommended before or in conjunction with implementing optimized maintenance models. If you are interested in learning more about how Credera can help you optimize your asset reliability and maintenance processes or about machine learning in general, please reference this blog post about machine learning use cases or reach out to us at marketing@credera.com.
Contact Us
Ready to achieve your vision? We're here to help.
We'd love to start a conversation. Fill out the form and we'll connect you with the right person.
Searching for a new career?
View job openings