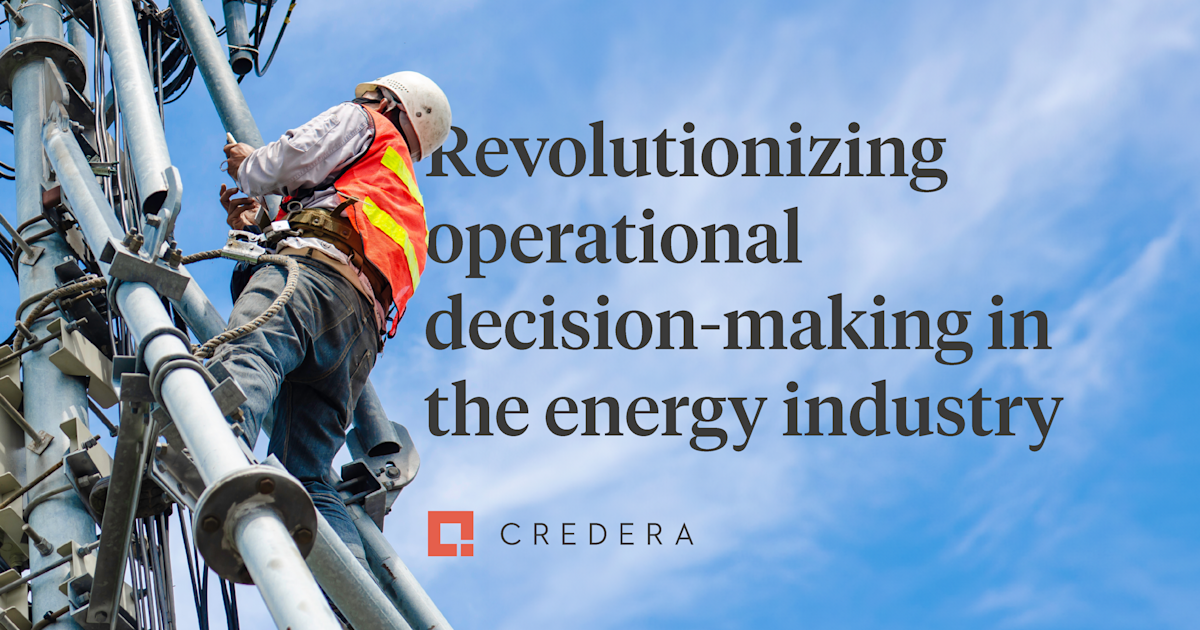
[The following is an excerpt from an ebook—download the ebook to read more.]
According to PR Newswire, 85% of business leaders have experienced decision distress, and 93% believe that the right decision intelligence is essential for organizational success.
That’s definitely true in the energy sector, where presenting the right data to the right decision-maker at the right time is critical. AI-powered solutions can make a significant difference, swiftly processing vast amounts of data and providing actionable insights that transform operations.
To do this successfully, the first step is to develop a framework that ensures AI tools are not using irrelevant data that confuse the operating picture—or creating the risk of an information security breach.
In this article, which is part 3 of our AI in the Energy industry series, we’ll take a look at Credera’s approach to this framework, which involves a technique for enhancing generative AI models called retrieval augmented generation (RAG).
Reduce the noise and uncover your specific value drivers with our AI Innovation Workshop.
Credera’s approach: Establishing a framework
In 2020, Patrick Lewis from Meta AI Research (formerly Facebook AI Research) and Ethan Perez from New York University introduced retrieval augmented generation (RAG) in their paper titled Retrieval-Augmented Generation for Knowledge-Intensive NLP Tasks. RAG combines the strengths of information retrieval and natural language generation to produce highly accurate and contextually relevant responses to questions.
Imagine a solution that not only understands your question but also pulls in the most relevant data from vast knowledge bases to give you a precise and actionable answer. That's RAG in action.
RAG techniques are designed to elevate the quality of responses, especially in specialized fields or when drawing from private knowledge repositories like company databases. This ensures that AI systems deliver information that is both factually correct and up-to-date.
Think of it as a smart assistant that when asked a question, seamlessly fetches and integrates the most relevant information to provide you with a clear and accurate response. This makes RAG an invaluable tool for decision-makers who need reliable and comprehensive information at their fingertips.
A typical RAG system consists of several key components that work together in a multi-step process. Here is a simplified overview:
Ingestion: In this initial stage, documents and other unstructured text content are processed—the content is extracted, chunked into manageable pieces, and converted into vector representations (embeddings). This step transforms raw data into a format that can be efficiently stored and searched.
Storage: The embedded representations of the data are stored and indexed, either in a vector database or a graph database, depending on the specific use case. This organized storage allows for quick and efficient retrieval of relevant information when needed.
User prompt: The process begins when a user submits a query or prompt. This is the starting point of the RAG system's operation and determines what information needs to be retrieved and generated.
Retrieval: Based on the user prompt, various search algorithms are applied to retrieve the most relevant content from the data sources. This step enriches (contextualizes) the user prompt with pertinent data and facts, ensuring that the most appropriate information is fetched to support the response.
Generation: The enriched prompt is passed to the large language model (LLM). The LLM uses this contextually enhanced prompt to generate a response, resulting in a coherent, contextually appropriate, and factually accurate text output. This final step combines the power of the LLM with the retrieved information to produce a comprehensive answer.
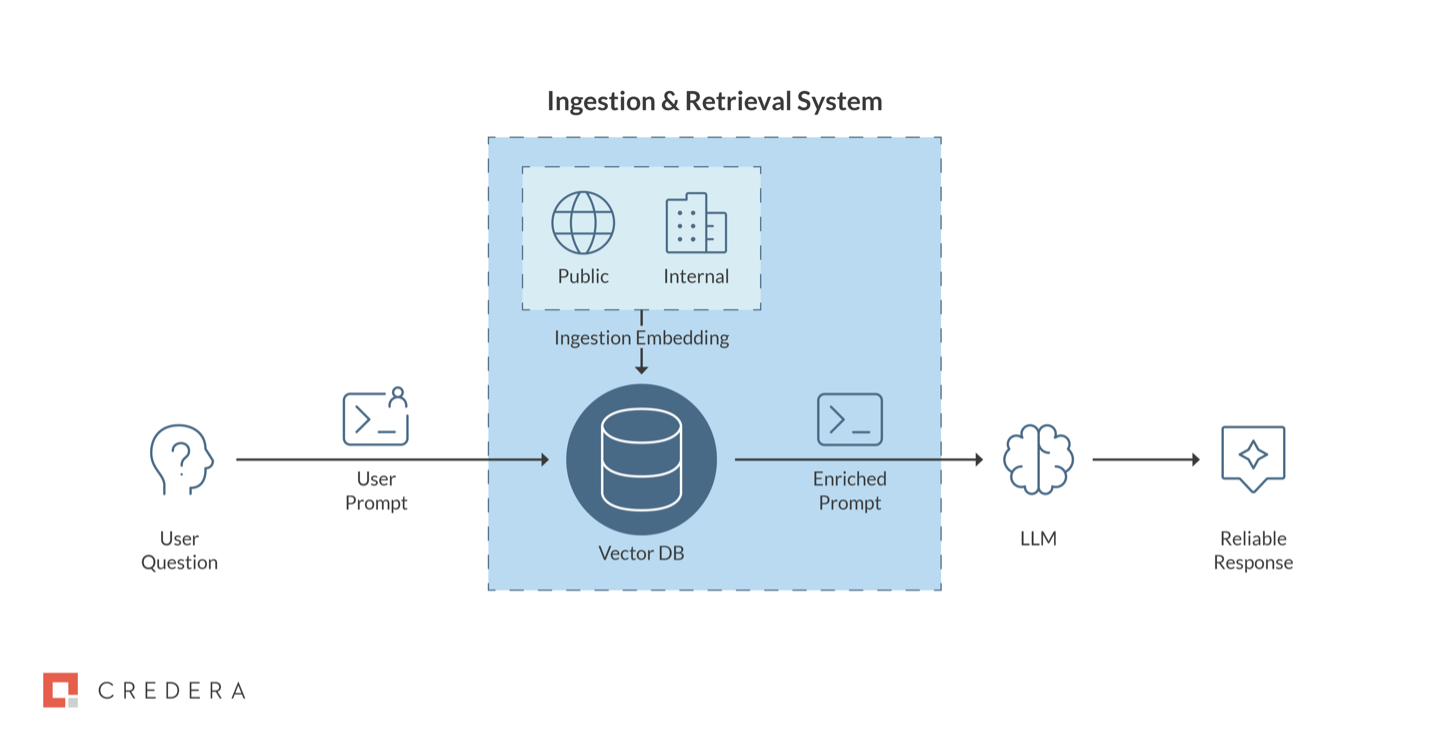
Following this process, RAG systems can provide responses that are not only fluent and coherent (thanks to the capabilities of large language models) but also grounded in specific, relevant information retrieved from the knowledge base.
Energy use case example: Risk mitigation for maintenance projects
To illustrate the practical application in the energy sector, let's consider a scenario focused on risk mitigation for an upcoming maintenance project where we’ve developed a Gen AI application that leverages RAG.
First, we will ensure that our application has access to a diverse range of relevant data sources, including:
Geographic location details of the project site
Projected timeframe for the maintenance activities
Historical safety incident reports from similar projects
Current and forecasted weather conditions for the project duration
With this information integrated into our application, we can now pose complex queries that traditionally require extensive manual research, analysis, and synthesis. For example:
"What are the primary safety concerns for our upcoming maintenance project, considering the location, timeframe, past incidents, and weather forecast?"
Our app will:
Retrieve relevant information from each data source
Analyze the combined data for potential correlations and risks
Generate a comprehensive, context-aware response
This approach offers several key advantages:
Efficiency: Eliminates the need for manual searching and curating of information from multiple sources.
Comprehensive analysis: Considers a wide range of factors simultaneously, potentially identifying correlations that might be overlooked in manual analysis.
Actionable insights: Provides concise, relevant information tailored to the specific query.
Rapid response: Enables quick decision-making based on up-to-date, synthesized information.
The bottom line
Our specialists have developed repeatable, actionable plans you can follow to quickly leverage the power of AI and drive efficiency and growth. Schedule a call with us to learn more about the full potential of AI in the energy industry and talk about enhancing your operational decision-making with RAG, and check out the rest of our content in the AI in Energy series here:
Contact Us
Ready to achieve your vision? We're here to help.
We'd love to start a conversation. Fill out the form and we'll connect you with the right person.
Searching for a new career?
View job openings