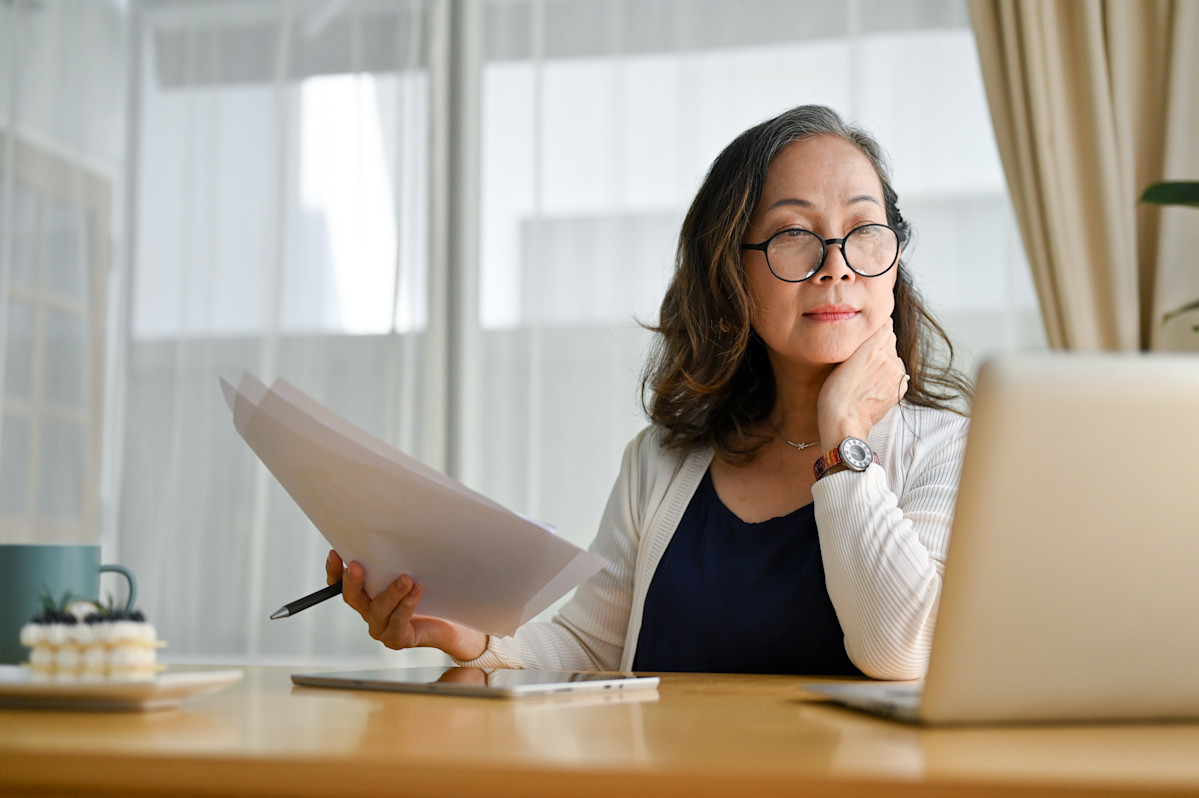
AI is all about simulating human intelligence. However, AI is a broad field with different levels of sophistication and capabilities. He threw light on the different types of AI:
Narrow AI may excel in specific tasks but lacks general intelligence. Examples include speech recognition systems, recommendation algorithms, and self-driving cars.
Artificial General Intelligence (AGI) can perform any human task. It demonstrates human-like intelligence and adaptability. Although theoretical, AGI does present a major milestone in AI research.
Super AI surpasses human intelligence and it does so in several aspects, raising significant ethical and societal concerns. This level of AI remains speculative but is a central point for discussions about the future of AI and its impact.
He explained the concept of machine learning (ML) and how it is a subset of AI which enables algorithms to learn from data and predict outcomes based on this learning. He spoke about machine learning and its two main types:
Supervised learning, where algorithms are trained on labeled data, meaning the input comes with the correct output.Unsupervised learning, where algorithms are given data without explicit instructions on what to do with it, aiming to find patterns and relationships within the data.
He then delved into deep learning and generative AI, both of which are driving significant advancements in AI technology today. The above understanding set the context for the core topic of prompt engineering
Prompt engineering
Prompt Engineering is a technique designed to optimize the performance of language models. By carefully constructing prompts, we can refine language model outcomes to enhance accuracy, coherence, and relevance. This approach extends benefits to tasks such as summarizing texts and answering questions.
Giving several examples, Gaurav highlighted the key elements of effective prompt engineering.
Understanding prompt architecture is critical as it involves guiding the model’s behavior and continuously refining prompts to improve outcomes. A well-constructed prompt ensures that the language model understands the desired task and produces the expected response.
For formulating effective prompts, Gaurav shared several strategies:
Write precise instructions: Clearly define what you want the model to do. For example, instead of a basic prompt like “generate an email reminding a customer about their abandoned cart,” an effective prompt would be: “Generate an email reminding [customer name] about the items left in their cart. Mention the specific products (e.g., stylish blue sneakers) and any limited-time offers and discounts.”
Define tone and style: Specify the tone you want the response to have. For instance, a basic prompt might be “write an email thanking a customer,” whereas an effective prompt would be “write a warm and appreciative email expressing gratitude to the customer.
Provide context: Give the model sufficient context to understand the task fully. This ensures the response is relevant and accurate.
Specifying the length of the outcomeIndicate the desired length of the response to prevent overly verbose or too brief outputs.
Chain of Thought (CoT) promps: Encourage the model to explain its reasoning process. This method, known as chain-of-thought prompting, can improve the quality of responses for complex tasks.
Prompt chaining: Break down complex tasks into a series of simpler prompts. This sequential approach can help tackle intricate problems more effectively.
Myths about prompt engineering
During the session, Gaurav also debunked several myths surrounding prompt engineering:
It is a one-time task: Prompt engineering requires continuous refinement and adaptation to improve model performance and meet evolving requirements.
Anyone can become a prompt engineer with minimal effort: While learning the basics is accessible, mastering prompt engineering demands a deep understanding of language models, continuous learning, and practical experience.
It is a guaranteed path to a successful generative AI project: Effective prompt engineering is crucial but not a magic bullet. Successful AI projects depend on various factors, including data quality, algorithm selection, and implementation strategies.
There’s a single perfect prompt for every task: Multiple prompts may be needed to achieve the best results, as different approaches can yield different outcomes
It eliminates the need for human oversight and bias mitigation: Human oversight remains essential to ensure ethical considerations, bias mitigation, and the responsible use of AI.
Gaurav highlighted several use cases of Generative AI in the Business Process Management (BPM) industry, showcasing its transformative potential:
Content generation: The creation of content can be automated for requirements such as marketing materials, reports, and emails, to enhance productivity and consistency
Process optimization: AI can be leveraged to analyze and optimize business processes, resulting in increased effciency and reduced costs.
Predictive analytics: AI can be deployed to predict future trends and outcomes, enabling proactive decision-making.
Customer engagement: Customer interactions can be enhanced through personalized responses and recommendations, improving customer satisfaction and loyalty.
Risk management: Risks can be identified and mitigated through predictive models and data analysis, helping businesses stay ahead of potential problems
Despite its potential, Generative AI also has limitations:
Data dependence: The quality and quantity of data directly impact the performance of AI models. Poor data quality can lead to inaccurate results.
Hallucinations: AI models can sometimes generate incorrect or nonsensical outputs.
Lack of common sense: AI models often struggle with tasks requiring common sense understanding and reasoning.Interpretability: The decision-making process of AI can be unclear, making it difficult to understand how conclusions are derived.
Potential for misuse: AI technology can be misused, raising ethical and security concerns.
Legal and regulatory challenges: The evolving landscape of AI regulation requires ongoing attention to ensure compliance and ethical use.
By embracing the art and science of prompt engineering, we can unlock the full potential of AI, driving innovation and excellence across industries. Gaurav Verma’s session has equipped us with the tools and understanding to navigate this evolving landscape, ensuring we remain at the forefront of AI advancements.
As we continue on our journey of learning, Prompt Engineering emerges as a critical skill set for AI practitioners. Credera remains committed to empowering individuals and organizations with the knowledge and skills needed to thrive in the digital era through sessions like these. Stay tuned for more instructive sessions and insights as we continue to level up our learning at Credera.
Contact Us
Ready to achieve your vision? We're here to help.
We'd love to start a conversation. Fill out the form and we'll connect you with the right person.
Searching for a new career?
View job openings