Data
Dec 22, 2020
Data Quality Part 2: The Scapegoat for Bad Data Quality and Why It’s Wrong
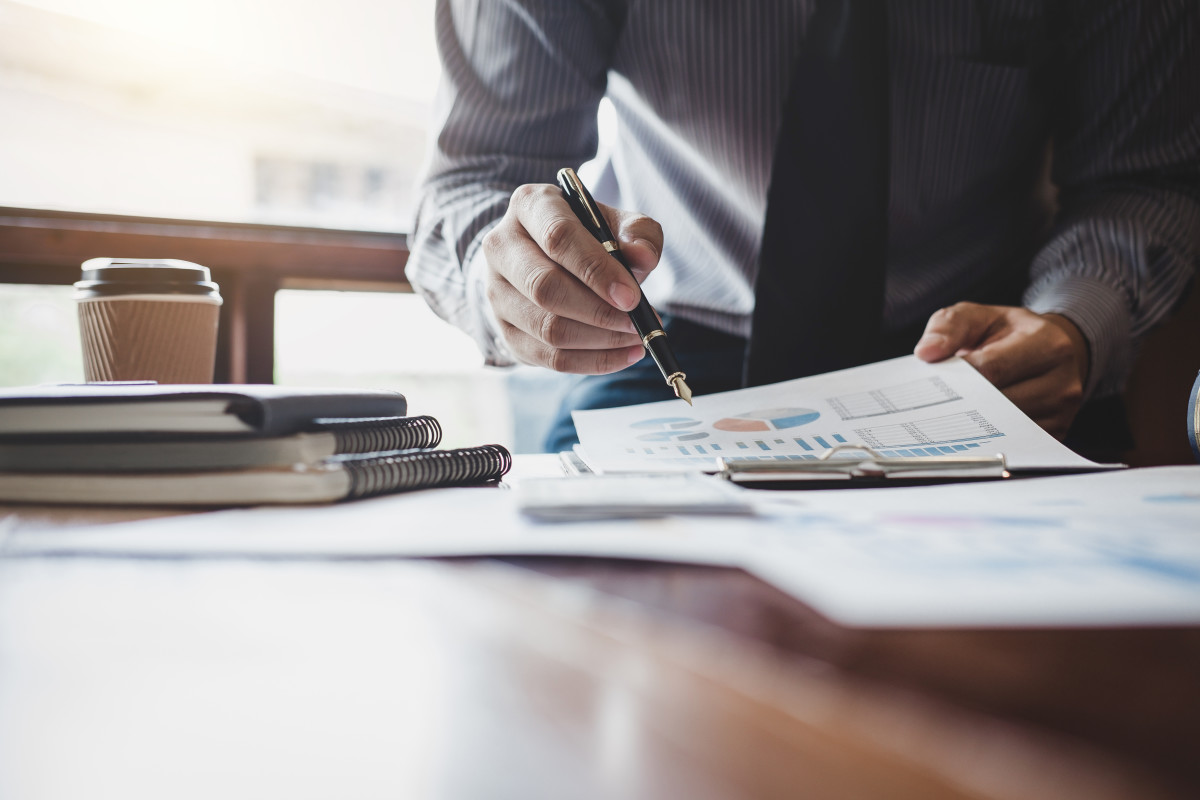
In the first blog post of our data quality series, we talked about the common data problems your company is most likely suffering from. Fixing those problems requires understanding what caused them, and that’s the next misstep. Too often companies blame complexity and scale when the real problem is a lack of data governance.
The Scapegoat: Organizational Complexity and Scale
When it comes to data quality issues, the complexity and scale of your organization are often blamed as the cause. You start with a simple data model, a handful of data marts perhaps, and a business intelligence (BI) reporting toolset to begin developing key performance indicators (KPIs), simple forecasts, and performance dashboards. As data use increases, more data is generated and collected and more data is consumed by various groups within your company.
It starts with organizations that are hungry for data, then leads to embracing loosely coupled systems, which leads to a duplication of effort, a burden on customers, and finally technical debt. As the need for more data grows, as more data is both ingested and used, the initial infrastructure built to handle demand begins to crack and legacy systems which were at one point state of the art are now creating headaches at several stages of your information workflow.
As technical debt grows in order to keep up with demand, the quality of data is diminished, and bad data starts to work its way into models and reports. As systems now ingest data from other systems and machine learning (ML) applications become more ubiquitous, the bad data is ingested without a human being even looking at the data before it’s reprocessed and inserted into another model downstream or worse yet, is presently directly to a customer through a recommendation or price for example. It’s no wonder the following statistics are true when it comes to data quality in organizations:
95% of companies believe they have inaccurate data
One in three business leaders don’t trust the information they use to make decisions.
Poor data quality costs the U.S. economy around $3.1 trillion annually.
Bad data quality is costing business 30% or more of their revenue.
Knowledge workers waste 50% of their time hunting for data, finding and correcting errors, and searching for confirmatory sources for data they don’t trust.
The Real Culprit: Lack of Data Governance
Complexity and scale aren’t the real causes but are often the scapegoats for most companies. Rather, a lack of data governance is often the culprit. Even in the largest systems, having good data governance in place can help keep data quality issues at bay and prevent data quality problems from forming as your data ecosystem grows in size and complexity. At a certain scale, a human-driven process is unable to keep up (especially when ML-based data ingestion is involved), which leads us to a different data governance approach. In other words, this is not your father’s data governance.
Continue the Data Quality Journey
As we’ve worked with clients over the years dealing with these problems, we’ve quickly discovered that the answer isn’t to scale down the system or rip and replace every system older than X number of years. Rather, it’s employing state-of-the-art intelligence and models to form a more effective toolset that can both keep up and weed out data quality issues before they have a chance to pollute other models or outputs.
In our next blog posts, we are going to discuss our approach to data governance followed by the components we leverage in data governance systems we build and use with our clients regardless of the size, age, or complexity of their data infrastructure. If you’re interested in talking about data governance at your organization, reach out to us at findoutmore@credera.com.
Contact Us
Ready to achieve your vision? We're here to help.
We'd love to start a conversation. Fill out the form and we'll connect you with the right person.
Searching for a new career?
View job openings