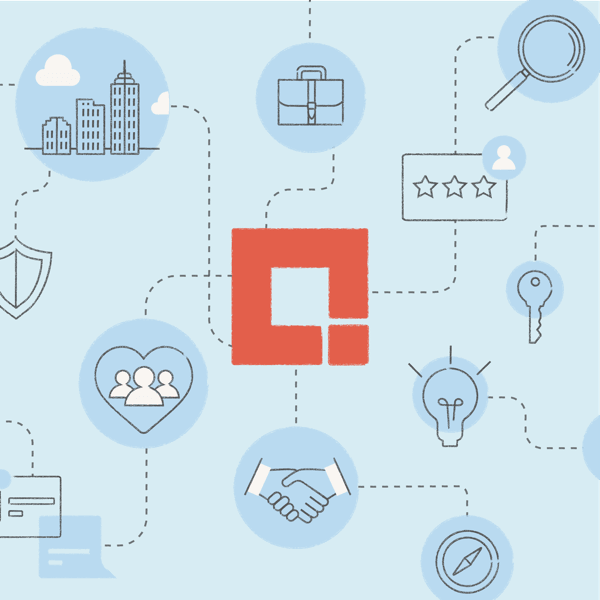
For those tired of seeing in the “Recommendations for You” section of their favorite online retailer a ‘Tickle Me Elmo’ doll based on a single purchase for their nephew five years ago, there is hope. In fact, now on my Amazon home page (as long as I don’t flush my cookies and sign out), the following categories are all personalized:
• New For You
• More Items to Consider
• Related to Items You’ve Viewed
• Get Yourself a Little Something
• Inspired by Your Browsing History
• Recommendations for you in MP3 Downloads
• Your Recent History
Again, we’re talking about only the home page. Any given product details page from Amazon then displays the following:
• Frequently Bought Together
• Customers Who Bought This Item Also Bought
• Customers Viewing This Page May Be Interested in These Sponsored Links
• What Do Customers Ultimately Buy After Viewing This Item?
What’s a guy to do with such a virtual cornucopia of personalized recommendations?! Better yet, what’s an online retailer to do with so many options…and so little time?!
Here are a few keys in determining the Recommendation Engine fit for your online needs:
Champions and Challengers
A simple search on the net shows a bevy of recommendation engines, most offered either as Software as a Service (SaaS) solutions or integrated into a larger eCommerce or report package. An initial separation of the champions and challengers, however, is…well…champion and challenger toolsets. Otherwise known as multivariate testing, several Recommendation Engine solutions have controls allowing head-to-head recommendation strategies, winner take all!!!
By having controls such as a percentage split of a current recommendation strategy to a new strategy test, Recommendation Engine tools allow a new strategy to ‘ease in’ and either prove or disprove itself. Much like Business Intelligence (BI) or broader Analytics environments, the smarts are usually in the people tweaking the tools, not the tools themselves. Still, a good Recommendation Engine should offer as many out-of-the-box recommendation strategies as possible in order to provide a solid baseline to further configure/customize to your heart’s, or OCD’s, desire.
The( Online) Island of Dr. Moreau
Just as H.G. Wells envisioned a place where man and animal could be genetically cross-bred to create new species, throwing in customer profile and purchase history with browsing history and promotions based on customer loyalty can be mixed up in multiple recommendation algorithms. Well, perhaps Dr. Moreau is a bit of a stretch, but throwing merchandising controls on top of a solid algorithm can help the horror of ‘Tickle Me Elmo’ quickly disappear from a customer’s future landing page.
Merchandising controls and recommendation algorithms should take into account (and be able to adjust ‘on the fly’) each of the following:
• Space and time information – yes, recommendations can be relative (thank you, Dr. Einstein) based on where a customer is located (gathered through geocoding) and what time it is based on where the customer is located. You’ve got to know when to recommend the Bay City Rollers singing “Saturday Night” on MP3, right?!
• Affinity or Collaborative information – essentially, affinity looks at what other people have done against what a customer who is like those other people is doing. Common affinity models are labeled “Customers who viewed this purchased this,” “Customers who viewed this also viewed this,” etc.
• Product or service information – for online retailers, merchandising is key, and recommendations looking at most sold, best conversion rates, most buying sessions, most viewing sessions, etc. go a long way in recommending “Best Sellers” which take into account product margin or current product promotions. Not to mention product packaging, including up- and cross-sell suggestions based on product type, description, price, margin, etc.
• Browsing behavior – the online version of the Cookie Monster isn’t a big, hairy, blue beast, but rather a tracking mechanism. What pages you’ve viewed in the past, what pages you’re viewing now, and the time you spent on each page is available to make recommendation decisions. And yes, it does feel a bit like Mr. Smith saying in The Matrix, “As you can see, we’ve had our eye on you for some time now, Mr. Anderson.”
• Customer information – the holy grail of recommendation data is the customer login, or authentication, with previous purchase history, loyalty data, demographic analysis, wish lists, likes, ratings, personal information and abandoned shopping cart contents available. If a loyal customer receives an email offering a discount off the abandoned product left in their shopping cart the day before, they might be persuaded to buy.
• Social information – beyond a ‘Likes’ tag from Facebook, and related to affinity information, social data considers a customer’s friends, a space which is rapidly evolving. Brand merchants can use community information to let a customer know what shoes their friend bought, pushing the same brand with an alternative style. You can even see commercials touting the purchase of new items based on following a celebrity’s style. Finally, a social maven’s dream world!
Mixing inputs, adjusting algorithms and testing groups should be an ongoing process. Many Recommendation Engine vendors go as far to guarantee a 5%-20% lift in online revenue based on the implementation of their Recommendation Engine. Year 1, sure! It’s the “long tail” of retail to be concerned with, tweaking formulas to constantly learn more about customer segments and successful recommendation strategies.
Domo Arigato, Mr. Recommendo
Thankfully, most Recommendation Engines come with strong analytical/reporting environments, and the best implementations utilize the environments daily. Conversions, average order value (AOV), impressions and clicks are obvious success metrics. Not so obvious is categorizing customers according not only according to profile but also buying vs. browsing behavior (and a bevy of other data inputs). Consider the following:
Customer 1: Married male, mid-40s, father of three children ranging from 4-12 years old
Customer 2: Single female, early 20s, recent college graduate
Let’s say you’re an online clothing retailer who gathered customers and profiles based on a paid marketing list and the two customers above ended up purchasing something from your site. You’ve now got demographic data (including their ship to address) and some buying behavior. Next, you target an email campaign to each consumer type:
Customer 1: Back-to-school special, noting an upcoming tax free weekend in the customer’s state
Customer 2: Women’s fall fashion promotion, including an online coupon
If each customer converts on the email campaign offer, there should be enough data for targeted, personalized recommendations – and here’s where online retailers make the ‘Tickle Me Elmo’ mistake:
Customer 1: First online purchase made on a weekday evening of a golf shirt; second order made in store of over a dozen children’s items on a Saturday afternoon, causing future recommendations to be heavily weighted to children’s clothing due to customer purchase history
Customer 2: First purchase made online on the East coast of trendy women’s shoes shipping to customer address on the East coast; second purchase made online on the West coast of two business suits shipping to a separate address on the West coast, causing future recommendations to be weighted toward cross-selling women’s fashion items due to customer browsing history
At this point, you can already see the problems with pre-set merchandising weights for these two customer examples. Specifically, example reweighting and missed opportunities are as follows:
Customer 1: Heavier weighting toward channel (online vs. store) and potentially time of day for recommendations can assume different customers and recommend different products; also, current session browse history rated highest can capture and recommend best what a customer is currently looking for, so as not to miss the new line of premium golf shirts for dad
Customer 2: Heavier weighting of geolocation and again, current session browse history can also assume different customers and recommend different products to perhaps up-sell the original East coast customer into buying more trendy products rather than cross-sell her mother on generic women’s fashion on the West coast
In summary, all online analytics should support evolutionary marketing/merchandising controls, continually testing, refining and recasting customer profiles against algorithm weights. Thank you very much, Mr. Recommenation.
Categorizing Differentiators
So far, we have the following key elements described:
• Multivariate testing
• Out-of-the-box recommendation strategies
• Recommendation algorithms
• Merchandising controls
• Analytical/reporting environments
Which can be added to the standard differentiators regardless of third party product:
• Architecture and SLAs
• Integration approach
• Implementation and upgrade strategy
• Product roadmap
• Pricing
With pricing in particular, keep in mind there are flat fee models based on impressions, click-throughs or site revenue, as well as revenue share models based on attributed revenue (via click-throughs) or incremental lift (above and beyond a forecasted amount). Your risk tolerance, site expectations and budget will play heavily in determining the right fit here.
An inept job candidate’s recommendation letter might read, “I most enthusiastically recommend this candidate with no qualifications whatsoever.” Similarly, it would be better to implement no recommendations at all than ignorant recommendations. Starting with the end in mind is indeed a highly effective habit!
Contact Us
Ready to achieve your vision? We're here to help.
We'd love to start a conversation. Fill out the form and we'll connect you with the right person.
Searching for a new career?
View job openings