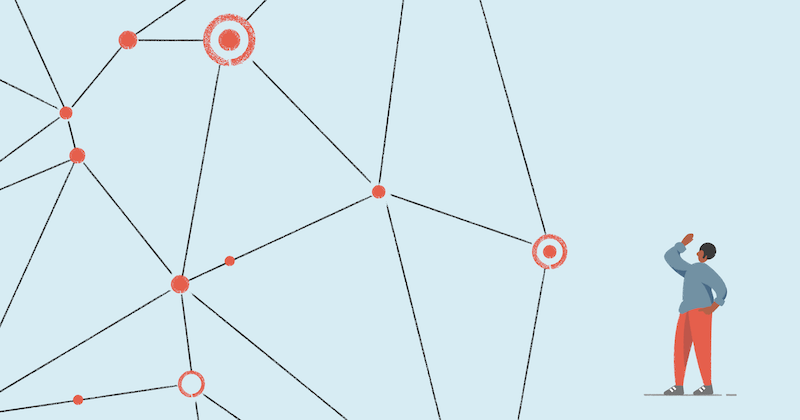
In this four-part series, we offer practical advice on how organisations can successfully realise value from data by running through the detail of our eight-layer data operating model.
In the first instalment of this series, we discussed the first two layers of our eight-layer cloud operating model: 'Organisation' and 'People & Culture.'
In this blog, we address the third and fourth layers: ‘Data Products and Services’ and ‘Data Governance and Performance.’
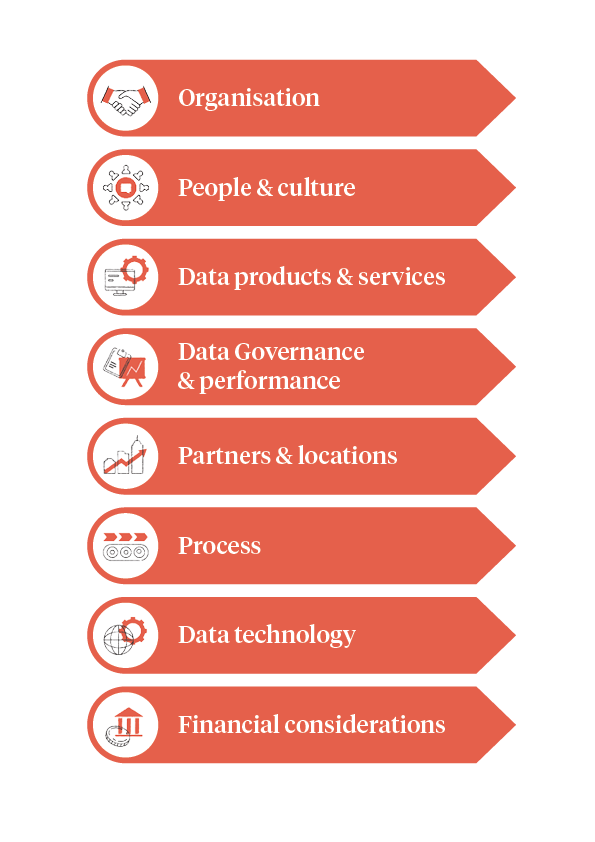
Read next: Transforming your organisation to succeed with data: Part three Data products and services

Understand customer needs and make them your priority
Data-driven organisations create and improve data products, enabling the organisation to access and analyse data from disparate sources, gain insight, and make decisions that take the organisation forward. Organisations seek to adopt a data product-centric approach as part of a data operating model using methodologies already applied to software product development. Gartner’s research states that 85% of organisations have either adopted, or plan to adopt, a data product-centric delivery model.
The move towards data products and the innovation surrounding new data-related technology and concepts (such as data mesh) need to be supported internally. This requires an integrated data operating model which considers the following data product characteristics:
Data products, designed for re-use and speed of delivery
Standard data product patterns are developed to be applied according to the particular process / treatment that the data needs to go through, and typically considers the stages of data ingest, transform, cleanse, catalogue, store, process, model, egress, analyse, consume, archived, and purged. Each time data requires a particular treatment, a standard pre-approved pattern is selected that meets that particular use case. Pre-approved, off the shelf, self serve patterns that support enhancement / tweaking can only serve to increase speed of delivery.
In a similar way, common services that are called upon by data products can be standardised and made available for rapid application. Examples of standard services include:
Data security
Monitoring, logging, and alerting
Secrets management
Data privacy
Access management
Data management
Service and discovery registry
Design and development (Source Control, Continuous Integration/ Continuous Deployment Pipeline)
Metadata
Data flow scheduling & management
Ingestion
Egress
Audit
Operations
Virtualilsation
Disaster recovery
Product persistence
Analytical environment and self serve platform
Delivering immediate value from data products
A product-centric approach means that dedicated data product teams can apply an Agile framework to the way they work. Associated roles, ceremonies, and principles can be applied that are focused on delivering value through sprints (Scrum) or continuous delivery (Kanban). Data product owners make decisions about how to maximise value from the product as it develops, taking feedback from data product end users, customers, and stakeholders to order the backlog.
Data governance and performance
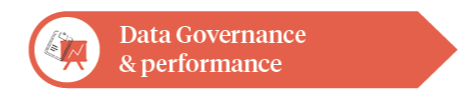
The data governance practice acts as a bridge between the IT teams and the business and should be set up to enable progress. Risks should be mitigated and value maximised without detriment to the delivery of data related programmes of work. The governance team will introduce and monitor the compliance of data policies, standards, and procedures by having data experts and stewards within each data domain frequently interacting with data producers and consumers. Using standard pre-approved patterns and standard services to develop data products should lead to a change in how governance is applied across an organisation. Governance should be seen as an enabler and not a blocker to delivery at pace.
Data domains
Each company should look across its enterprise to sensibly divide the organisation into four-to-eight data domains. These domains are logical data groupings that can enable specific policies and procedures to be applied, making governance simpler and more directive.
Having data domains identified and implemented allows the data governance team to apply and monitor frameworks that span across across disparate IT systems. This will help to ensure that data is managed in the same way. Within a 'customer' data domain, for example, it is known that the domain will contain personal identifiable information (PII) and it is therefore to be expected that additional security and identify access management (IAM) policies will need to be applied to all platforms and warehouses where this data is stored. In comparison a finance data domain, may require a different IAM policy.
Additionally, each data domain will have one 'golden record' of data which brings data together from different lines of business, and supports with aspects such as creating a single view of the customer. This is where all data is mastered and cleansed, and has specific data quality rules applied. The data governance team will work with the architecture team to ensure that this is best placed within the data lifecycle for each domain.
Critical data governance roles
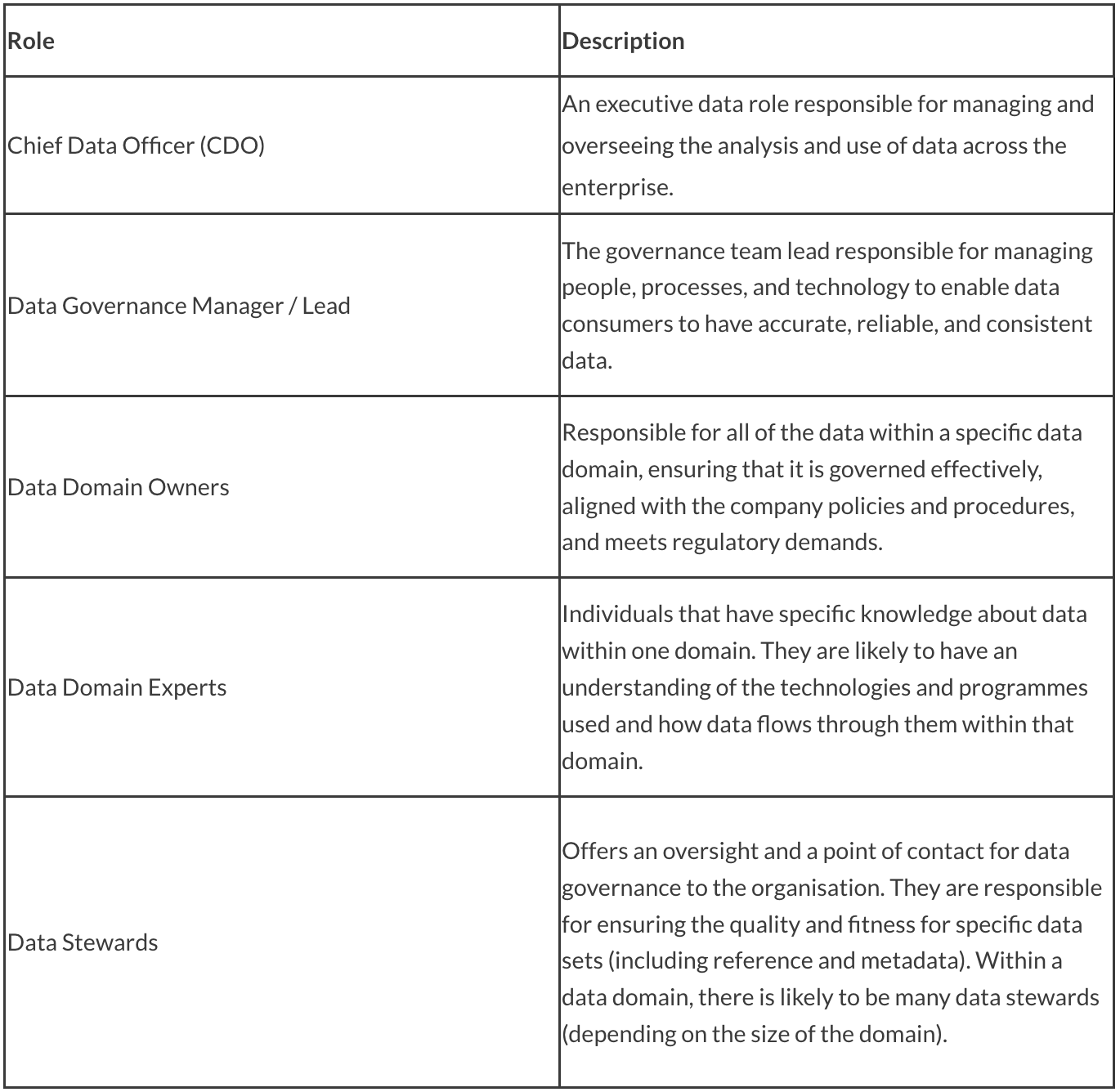
Data tooling
Data management tooling facilitates data management activities and ultimately aims to improve the performance of master data, metadata, and reference data. The tools often use metadata to enable the visualisation of data lineage, hold the organisations data dictionary and taxonomy, catalog data, capture data quality rules and visualise performance, and store data privacy policies. Data management tools are owned by the data governance manager at a central location of the business, and are primarily updated by data stewards.
Data quality metrics
Data quality metrics cover seven dimensions (including; accuracy, completeness, consistency, integrity, reasonability, timeliness, and uniqueness) that run through all golden records in each data domain. These are essential to enable not just the data governance team on where data issues need attention, but also the data consumers to identify the quality of the data that they are using in their data products. As data metrics are meant to be accessed across a wide range of data users, they need to be largely self explanatory and visual to ensure that they are principally understandable. Individuals should be clear on what is being measured and the measurement being used.
Data councils & forums
Once roles and practices have been established, a central reporting structure needs to be embedded to enable issues, metrics, and projects to be raised, discussed, escalated, and acted upon. A regularly occurring forum is established in each data domain which is chaired by the data domain owner and allows the data stewards to raise concerns on the use, availability, and/or quality of the data sets they oversee. Each data domain owner has an escalation route at a recurring data council that is chaired by the Chief Data Officer to raise concerns over data issues, security, and ask for funding to improve data services to the business. At both a forum and council level, metrics and KPIs are assessed to ensure the data practice is supporting the overarching business goals.
In a nutshell
Organisations should consider adopting a data product-centric approach as part of a data operating model. They should use methodologies already applied to software product development with common patterns, services, and Agile ways of working applied to realise value from data at speed.
Governance should be seen as an enabler and not a blocker to delivery at pace with clearly defined data related responsibilities.
Organisations should also consider the identification of data domains to ensure that data is managed consistently across disparate IT systems.
Contact Us
Ready to achieve your vision? We're here to help.
We'd love to start a conversation. Fill out the form and we'll connect you with the right person.
Searching for a new career?
View job openings